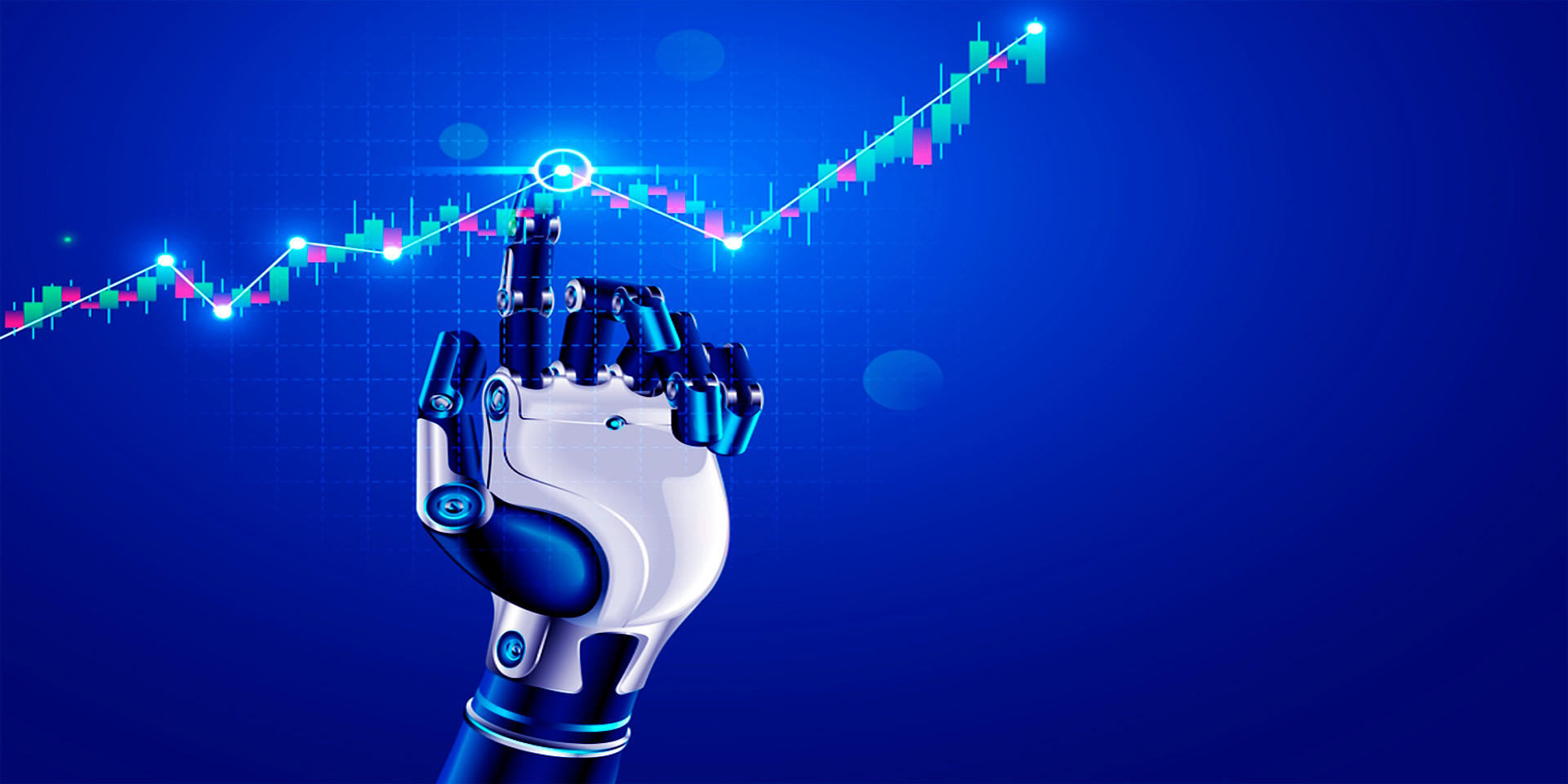
Leveraging Machine Learning and Best Practices for Robust Credit Risk Models
Machine learning technologies can revolutionize credit risk modeling by significantly improving accuracy and adaptability. These technologies handle vast datasets, uncovering patterns that traditional methods might miss, leading to better risk segmentation and prediction. Real-time decision-making becomes possible as AI continuously monitors and analyzes data, detecting early signs of default or risky behavior. Furthermore, machine learning models offer scalability and flexibility, adapting to changing market conditions and regulatory requirements. To build robust risk models, define clear objectives, ensure data quality, and use automation and sensitivity analysis. By leveraging these technologies and best practices, financial institutions can enhance their credit risk management and make more informed lending decisions.
How Can Machine Learning Technologies Enhance Credit Risk Modeling?
Machine learning technologies can enhance credit risk modeling by significantly improving prediction accuracy. By handling large datasets, you can uncover hidden patterns that traditional methods might miss, leading to better risk segmentation. This precision allows you to identify varying levels of risk among borrowers more accurately.
Another advantage is real-time decision-making. By continuously monitoring data, like transaction histories and social media activity, you can detect early signs of default or risky behavior, reducing your financial exposure. This proactive approach is essential for maintaining a stable financial environment.
Machine learning models also offer scalability and flexibility, ensuring your risk assessments adapt to changing market conditions and regulatory demands. This adaptability keeps your models current and effective, even in fluctuating environments.
Additionally, ML helps manage non-linear relationships in data, enhancing your ability to make accurate forecasts. By incorporating various data sources, you can gain a multifaceted view of borrower risk.
For practical application:
- Start by gathering and preprocessing data from both traditional and alternative sources.
- Use feature engineering to identify impactful variables.
- Utilize ML algorithms to analyze these variables to forecast default probabilities more effectively.
In the end, machine learning technologies can transform your credit risk modeling by improving accuracy, enabling real-time decisions, and providing scalability, ultimately leading to smarter lending decisions and reduced default risks.
What Best Practices Should Be Followed For Designing Robust Risk Models?
To design robust risk models, you should follow these best practices:
First, define clear objectives. Identify whether you want to manage financial risks, operational risks, or a combination. Clear objectives guide your analysis effectively.
Second, ensure data quality. Use reliable, representative data and regularly update it to reflect current conditions. This data should be accurate, complete, consistent, and relevant.
Next, collaborate with a diverse team. Involving bankers, data scientists, quants, and industry experts leads to more comprehensive models, combining various expertise.
Then, balance model design and complexity. Avoid models that are too simple or overly complex. Simple models may miss critical dynamics, while complex ones can be impractical and overfitted.
You should also stress test your model under various extreme scenarios to identify weaknesses and make necessary adjustments.
Regularly validate and back-test your model using historical data. Adjust parameters based on results to improve predictive accuracy.
Additionally, implement strong governance policies and internal controls to manage model risk effectively. This includes continuous monitoring and independent reviews.
You can use automation to enhance efficiency in model validation and operations, helping maintain consistency and reduce errors.
Conduct sensitivity analysis to understand how different variables impact outcomes. This can help refine and ensure your model’s robustness.
Finally, continuously monitor and recalibrate your model to adapt to new data and changing market conditions, ensuring that it remains relevant and accurate over time.
As a final point, by defining clear objectives, ensuring data quality, collaborating with experts, balancing complexity, conducting stress tests, validating models, implementing governance, using automation, performing sensitivity analysis, and ongoing monitoring, you can build reliable and insightful risk models.
How Do Financial Institutions Manage Risks Using Ai/Ml Models?
Financial institutions manage risks using AI/ML models in various powerful ways. You can rely on AI for fraud detection as these models analyze large datasets to spot unusual transactions, helping reduce fraud and money laundering risks. AI also aids in compliance management by monitoring transactions for suspicious activities and ensuring accurate reporting, keeping your institution compliant.
For credit risk assessment, AI enhances the accuracy of credit scoring and risk evaluations, making your lending decisions more reliable. You can also boost operational efficiency with AI, as these models automate data aggregation and model updates, streamlining your risk monitoring and decision-making processes.
Market risk analysis is another area where AI excels. It processes complex market data much faster than traditional methods, enabling your institution to respond quickly to market changes. Additionally, robust Model Risk Management (MRM) frameworks ensure your AI models perform reliably, including model validation and stress testing.
Bringing it all together—by leveraging AI/ML models, you can enhance fraud detection, compliance management, credit risk assessment, operational efficiency, market risk analysis, and model reliability, ensuring a more secure and efficient operation.
What Challenges Do Banks Face In Adopting Modern Risk Management Practices?
Banks face several challenges when adopting modern risk management practices.
First, you might be dealing with outdated legacy systems. These old technologies can’t handle modern risk management tools effectively, and upgrading them is costly and time-consuming. Then, there’s the issue of data management. Financial data is typically siloed, making it difficult for you to get a complete picture of risk, resulting in inefficient risk modeling and decision-making.
You also have to keep up with increasingly complex regulations. These regulatory demands require more sophisticated risk management, which can be difficult to implement. Finding and retaining skilled professionals who can navigate modern risk management practices is another significant challenge you might face.
In adopting new technologies, you might encounter operational risks like security breaches and system failures, which necessitate robust internal controls and continuous improvement. Market risks also pose a challenge as you need to adapt to market fluctuations and economic uncertainties, demanding advanced strategies to mitigate potential losses.
Integrating risk management with your overall business decisions requires significant organizational change, which can be tough. This integration is crucial for ensuring that risk management aligns with your business strategies.
All things considered, addressing these challenges involves investing in modern technologies, improving data management, and ensuring regulatory compliance, helping you enhance risk management and stay competitive in a rapidly evolving market.
How Can Financial Models Help In Evaluating Different “What-If” Scenarios?
Financial models help you evaluate different “what-if” scenarios by simulating the impact of various changes on your business. You might want to see how a 10% increase in sales or a sudden rise in production costs will affect your profits. By adjusting these inputs in a financial model, you can predict outcomes and make informed decisions.
Here’s how:
- Visual Representation: You gain a clear picture of possible financial futures, which helps you plan and strategize better.
- Risk Assessment: You can identify and understand potential risks and rewards by evaluating different scenarios, such as market changes or operational shifts.
- Data-Driven Decisions: Make strategic choices based on solid data rather than guesswork. Decide whether to expand or cut costs depending on predicted outcomes.
- Resource Optimization: Adjust your operations to optimize resources and improve efficiency under various conditions.
Financial modeling combines accounting, business insights, and technology to make these predictions. By simulating different scenarios, you can prepare for various possibilities and navigate uncertain futures confidently.
Lastly, financial models empower you to visualize outcomes, assess risks, make data-driven decisions, and optimize resources, helping you navigate uncertain futures confidently.